HonestyMeter - A Free Open Source Framework for Bias and Manipulation Detection in Media Content
"By embracing HonestyMeter, you can join the vanguard of a movement that champions media objectivity and transparency. The more people who adopt this tool, the more we can create a well-informed society where the truth prevails over bias and misinformation" Read the full article in MTS
Introduction:
Understanding HonestyMeter Through a Joke
The simplest way to illustrate what HonestyMeter addresses can be demonstrated through this joke: Upon his arrival in Paris, a reporter asks the Pope for his opinion on the city's famous bordellos. Surprised by the question, the Pope responds, "Are there bordellos in Paris?" The next day, the headline in the newspapers reads: "The Pope's First Question Upon Arrival in Paris: Are There Bordellos in Paris?"... Although the facts presented are 100% true, the way they are reported is 100% misleading. Even if the article provides full context, most readers read only headlines and will never know the details.
Truth Distortion
This anecdote underscores the type of misleading factual representation that HonestyMeter is designed to address –true statements framed in a context that can completely distort their intended meaning. This distortion is often achieved through sophisticated manipulation techniques such as sensationalism, framing, selective reporting, and many others, which can be applied either intentionally or unknowingly. These tactics can lead audiences to form distorted perceptions of reality, hindering their ability to make well-informed decisions. HonestyMeter aims to detect and clearly expose these tactics, assisting journalists in creating more objective content and empowering audiences to make better-informed decisions.
Why Manipulative Reporting is More Dangerous Than Fake News
It's important to emphasize that manipulative reporting is a much more dangerous phenomenon than fake news. False facts can usually be easily detected, and authoritative sources conduct thorough fact-checking before publishing any content, as publishing false facts leads to immediate accountability. Consuming news from credible sources can almost fully protect people from fake news. However, when content is published by an authoritative source and all the facts are real, but are presented using sophisticated hidden manipulation techniques, it can dramatically distort the perception of these facts. As demonstrated in the earlier joke, this kind of distortion can often lead the audience to understand something completely opposite from the truth, effectively equating it to fake news. Meanwhile, the source of this distortion typically faces zero accountability!
Introducing HonestyMeter: A Tool for Enhancing Media Objectivity and Transparency
To address this issue, we have developed the HonestyMeter framework – a free, AI-powered tool designed to assess the objectivity, bias, and manipulations in media content. Utilizing neural networks and advanced language models, HonestyMeter meticulously analyzes various media elements to identify potential manipulative tactics. It generates a comprehensive objectivity report, which includes an objectivity score, a list of detected manipulations, and recommendations for mitigating bias within the text. Wide adoption of HonestyMeter is capable of enhancing media transparency and objectivity worldwide, empowering authors to craft more objective content and enabling audiences to make better-informed decisions.
What Sets HonestyMeter Apart in Media Analysis?
Specialized Focus on Manipulations in Factual Information Presentation
Unlike basic fact-checking and bias/sentiment analysis tools, HonestyMeter focuses on sophisticated media manipulations. It detects how factual information is presented in misleading contexts, including the use of omission, framing, misleading headlines, and other similar techniques, which can lead to significant distortions of reality.
Free and Open Source
It offers cost-free access and its source code is publicly available, promoting transparency, wider accessibility, and community-driven enhancements.
Self-Improving System
HonestyMeter harnesses both AI and user feedback, continually refining its capability to identify and analyze media manipulations.
These features establish HonestyMeter as a unique entity in media analysis, addressing complexities beyond the scope of typical media analysis tools.
Features:
Our initial release focused on a singular feature, allowing users to copy text and receive a bias report. Below are the newly added features we have released in the past few months:
News Integrity Feed (New Release): Offers analysis of the latest news from leading sources. Users can search by keyword or filter by category and country.
Personal News Integrity Feed for Popular People (New Release): Analyzes the latest news about famous people. Users can search by name
Ratings (New Release): Features ratings for the most praised and criticized people, located on the "People" page, and ratings for the most objective sources, available on the homepage.
Custom Content Analysis (New Release - now with Link Support): Users can submit links or text to receive a comprehensive bias report. This feature enables analysis of content not featured on our website and allows authors to reduce bias in their original content.
Honesty Badge (New Release): Users who share our vision of transparent, unbiased media can display our badge alongside any content they post on platforms or social networks they manage or use. This enhances trust and engagement with the content. Each share promotes media transparency awareness, contributing to a fairer world.
There are three types of badges:
General Badge - Demonstrates support for transparent, unbiased media. Can be used with any content, anywhere.
Fair Content Badge - For authors or publishers of content that has achieved a high objectivity score and wish to highlight the objectivity of their content.
Medium and High Bias Badges - For publishers who wish to openly indicate the bias level in their content, thereby demonstrating extreme transparency. These badges are used in conjunction with the Fair Content Badge.
Auto-Optimization Based on User Feedback (New Release): This feature transforms HonetyMeter into a self-optimizing system, utilizing a blend of AI bias 'experts' and user feedback. Users have the ability to click on any section of the bias report and submit their feedback. This feedback is then reviewed by the AI. If the feedback is accepted, the report is updated accordingly, and the data is utilized for training and enhancing the model, thereby enabling continuous improvement in the accuracy of the reports.
Current State and Updates:
Over 18,000 reports generated.
Hundreds of new reports added daily.
Extensive coverage for each of the most popular people, e.g., over 500 reports on Elon Musk, Donald Trump and Taylor Swift among others.
Over 140 links from multiple websites in various languages, including listings and upvotes in leading AI tool indexes.
Surprisingly, HonestyMeter is used in multiple languages, despite being primarily English-focused.
The current version is an experimental demo. We're developing a more sophisticated version with higher accuracy and consistency. Nonetheless, even in its current form, HonestyMeter often provides insights difficult for humans to detect.
Technical Details
Evaluation Process:
The HonestyMeter framework uses a multi-step process to evaluate the objectivity and bias of media content:
Input: The user provides a link to media content, which may include text, images, audio, or video. (Currently, we support only text but plan to add more modalities in future versions).
Analysis: The framework uses large language models to analyze the media content and identify any manipulative techniques that may be present. The analysis includes evaluating the tone, sentiment, and language used in the content.
Scoring: Based on the analysis, the framework provides an overall objectivity score for the media content on a scale of 0-100. Additionally, the framework scores the objectivity level for each side represented in the content.
Reporting: The framework generates a report summarizing the analysis, scores, and feedback provided for the media content.
Feedback: The framework provides feedback to the user on the manipulative techniques identified and the areas of the content that may be biased or lacking in objectivity and suggests possible improvements.
Improvement: The user can take the feedback provided by the framework and use it to improve the objectivity of the content.
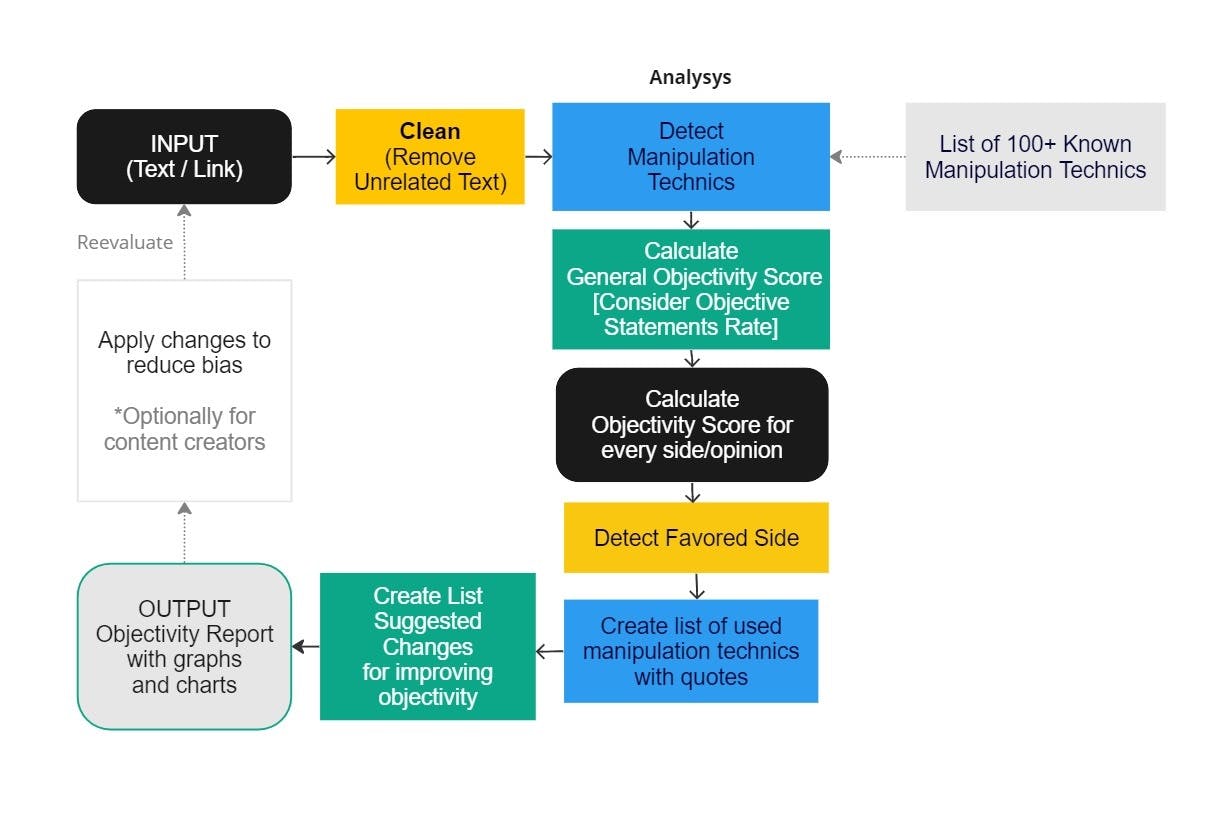
Example HonestyMeter Report Screenshot:
(GPT-4 Generated Article Explores Imaginary Debates Between Fictional Candidates in a Hypothetical Country)
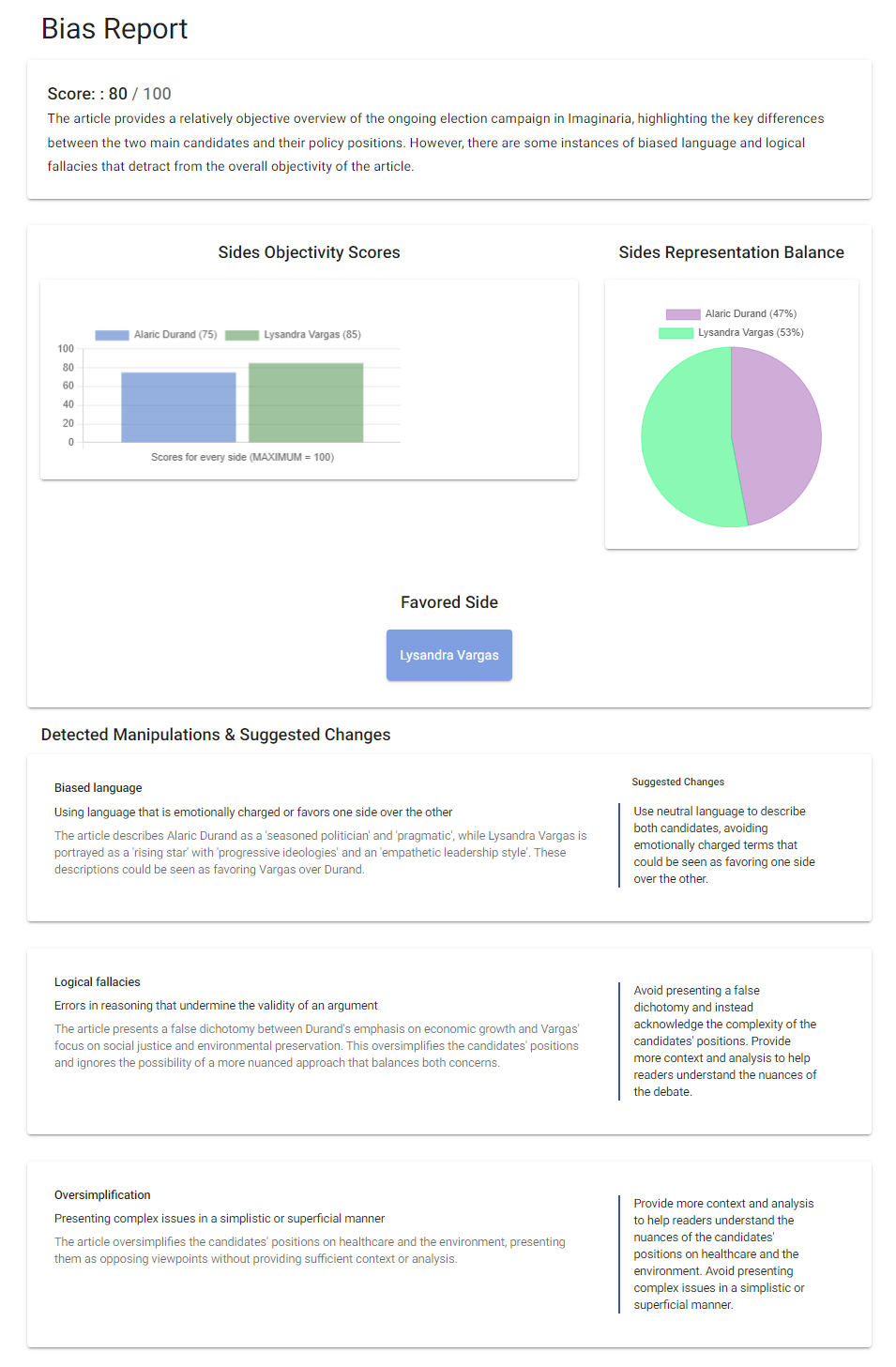
Technical Challenges and Solutions
The current version of HonestyMeter is an experimental demo. There is significant room for improvement in terms of the depth, accuracy, and consistency of the reports, for the following reasons:
- The GPT-3.5-Turbo model was used in production until the end of December 2023 to minimize costs, as the tool is free and self-funded. At the end of December 2023, we switched to GPT-4, reducing the daily report count as an experiment to prioritize quality over quantity.
- Large Language Models (LLMs) may face challenges in maintaining context in extended texts.
- In tasks that are complex and multi-stepped, LLMs tend to be less efficient, especially with lengthy inputs.
Therefore, we are actively developing more sophisticated, multi-staged algorithms to significantly enhance the reliability and consistency of evaluations.
Nevertheless, even in its current state, HonestyMeter frequently provides valuable insights that are challenging for humans to detect.
To enhance the results, we are undertaking the following steps. which are part of ongoing research and have not yet been fully implemented in production.
- We conducted in-depth research on manipulation techniques, gaining a comprehensive understanding of manipulation categories. We revised the list of manipulations and created a more concise and well-structured list that covers all manipulation groups without redundancy or omissions.
- We are moving away from our initial approach of relying on the LLM's "magic" with broad instructions, which was intended to prove the concept and showcase its potential. We are now working on providing the LLM with thorough, step-by-step instructions for detecting each manipulation technique.
- We broke down the evaluation process into the smallest possible tasks. We are currently testing distinct services for each micro-step, which involve chains of prompts, autonomous agents and individual models that are specifically trained and fine-tuned for certain tasks. This approach is anticipated to not only improve output consistency but also reduce the system's dependence on any single model and simplify the process of replacing existing models with better or more cost-effective open-source alternatives when necessary.
- We are experimenting with the most advanced Large Language Models (LLMs) and closely monitoring their exponential progress. By incorporating upcoming, newly released advanced models into our workflow, we expect to achieve significant enhancements in each component of our system, thereby leading to an overall elevation in performance.
- We have planned the release of seven versions of the app, each building upon the previous one and gradually incorporating more complex techniques for detecting manipulation. The first MVP version focuses on the most common and easily detectable manipulation techniques, providing a solid indication of bias levels. Every subsequent version builds upon this foundation, gradually adding more complex techniques for detection. The last three versions focus on the most advanced and complex techniques, offering the most detailed and thorough analysis of bias.
- We have added a user feedback feature. Unlike all the features listed above, this one is already released. User feedback is utilized to enhance our bias reports and to train our future models, turning our system into a continuously self-improving entity.
Feedback-Based Optimization Loop:
- A user who views the bias report clicks on the section that, in their opinion, should be changed.
- The user leaves feedback, explaining the suggested changes.
- The feedback is reviewed by a Large Language Model (LLM), following strict rules. To make only justified changes that improve or fix inaccuracies.
- If the feedback is accepted:
- The LLM updates the original report.
- The updated report is saved and used in the training dataset.
- The LLM is periodically retrained using the updated dataset.Resulting in constant improvement.
It's important to note that even with the current simplified experimental evaluation method, focused user feedback can lead to a more efficient revision of the report by AI. Presently, the task of creating the initial report is extremely challenging for LLMs, as it requires multi-step reasoning with large contexts.
However, if a user points out a very specific issue that should be reevaluated, it makes the task much easier, significantly increasing the evaluation's efficiency. If, as a result of user feedback, the report is amended by the LLM, it means that the specific part highlighted by the user is now more accurate than it initially was. Therefore, the revised report will be included in the training dataset.
Adhering to this approach of user feedback, LLM reevaluation, and training dataset enrichment empowers the system to autonomously enhance its capabilities, even without adopting the other upgrades listed above.
Accuracy, consistency, and deterministic outputs.
It's important to note that as long as the system correctly identifies the broad objective or manipulative nature of an article in most cases, it can provide statistically valuable insights into bias and manipulation levels, even if report accuracy and consistency are not perfect. This can be achieved by analyzing large volumes of content and calculating average scores from multiple iterations over the same articles.
For instance, by analyzing multiple articles from several sources and repeating the analysis of each article multiple times, we can identify which sources are more or less biased relative to each other. This approach, even with relatively low analysis accuracy, can yield statistically high confidence in the results.
Consequently, the effectiveness of the system isn't a binary choice between perfect operation and complete failure. It involves attaining a minimum required level of performance, followed by gradual improvements towards maximum effectiveness.
We are optimistic that through ongoing research and development, the efficiency and capabilities of HonestyMeter will steadily improve, gradually approaching its maximum potential. Even now, in its experimental demo phase, HonestyMeter frequently provides insights that are difficult for humans to discern.
Analysis Objectivity Verification: Overcoming LLM Biases
We developed a method to diminish evaluator bias, effective for both human and LLM evaluators, through content obfuscation. In our experiment, we replaced all recognizable entities in the content, including names, countries, political parties, and organizations. For instance, in place of debates between Biden and Trump, the obfuscated article discussed Rajish and Anil as candidates for chairman of a student organization in an Indian university.
The obfuscated content is particularly challenging for LLMs to recognize. To ensure the LLM could not identify the content, we explicitly prompted the LLM, explaining the obfuscation mechanism and asking the model to guess the real characters of the article.
After confirming the LLM's complete failure to recognize the actual characters and entities, we generated two reports using HonestyMeter: one about the original article and the other about the obfuscated article.
In all cases, the results were identical, proving that an LLM, when explicitly instructed to conduct neutral analysis, is indeed capable of a high level of neutrality, compared to humans.
Monetization in Harmony with Free and Transparent Media Integrity
Our main goal is to provide free, objective analysis based on a publicly open and transparent methodology. Currently, we self-fund the project by investing our own time and money, while offering it completely free of charge. We have received reasonable feedback from some users who noted that attracting additional funds could significantly aid in advancing the development and creating mass adoption. However, attracting substantial funds for a free service, based solely on social impact, without monetizing it and without offering any profit potential to investors, may prove challenging.
Therefore, we have created a list of possible monetization strategies that can work while fully retaining our vision of a free, open framework that makes the media more truthful and transparent. Implementing these strategies may help us in two ways: funding the project independently and attracting additional investments.
This list includes innovative products with unique commercial value, capitalizing on our core functionality. These products are targeting rapidly growing multi-billion-dollar markets, where even a minuscule market share could yield multimillion-dollar revenues.
- Honesty Badge and Commercial Content Analysis (unique value)
Honesty Badge Certification (currently available for free): A service that awards the 'Honesty Badge' to any content that meets high standards of objectivity and a low level of bias and manipulation. Optionally, every piece of content can be marked with an Honesty Badge showing its bias level: high, medium, or low to demonstrate full transparency.
Note: The high-end tier of this service may be provided in combination with human bias detectors and industry experts in niche relevant to the promoted content.
Target Audience: Any commercial company with a product or service, news portals, social networks, niche content blogs, and channels.
Honest eCommerce (in development): A content portal with highly objective commercial content.
Market Projections
The global content marketing market was valued at $407 billion in 2022 and is projected to reach $1.3 trillion by 2031, growing at a CAGR of 13.17%. (Source: Business Research Insights)Market Share 2023 Revenue Projection 2031 Revenue Projection 1% $4.5 billion $13 billion 0.1% $450 million $1.3 billion 0.01% $45 million $130 million 0.001% $4.5 million $13 million - Ads and Affiliate Links
Ads on Website: Revenue from website advertisements.
Affiliate Links: Placement of affiliate links within bias reports linking to news websites. Many news websites offer premium subscriptions and other products, which could potentially generate referral income. Currently, all links are regular, and even if a user coming from our link purchases a service, we don't get any commission. It can be easily changed if we decide to use this monetization method.
Market Size: Multimillion-dollar market with significant affiliate revenue. API Services
Analysis and Data Services: Suitable for publishers, news API providers, and researchers.
As part of operating our website, we create and save hundreds of bias reports about the latest news articles every day. These reports enhance the general news integrity feed on the homepage and contribute to a popular people integrity feed on the people page. In addition, we generate periodic ratings for the most praised and criticized individuals, as well as for the most objective sources, using an openly explained methodology.
Recently, we realized that gathering large amounts of data, enabling complex aggregations, and data analysis opens up potential avenues for future monetization opportunities.
API marketplace market size: was estimated at USD 13.74 billion in 2022 and is expected to grow at a compound annual growth (CAGR) of 17.8% from 2023 to 2030. ( Source: Grand View Research)- Other services and products based on HonestyMeter core technology
Honest News Portal: A subscription-based objective news service where the news is rewritten to present only neutral facts without bias or opinions.
Premium Features: Custom report and advanced database search for commercial use.
Additional Services: Including Email and Chat Analysis, Rewriting Service for Enhanced Objectivity, Video and Voice Meeting Analysis, and Chrome Extension as a Freemium bias analysis report generator.
Market Potential: Multi-billion dollar potential in fields like digital marketing, journalism, academic research, corporate communications, and content verification.
As shown in the table above, securing even 0.001% of the market, which currently amounts to $4.5 million, would be sufficient to sustain HonestyMeter's operations. By 2031, the market size is expected to grow, making 0.001% of the market worth an estimated $13 million.
Additional Monetization Options
Monetization Strategies Summary
The brief overview of monetization options illustrates the feasibility of combining free bias detection and a transparent methodology with various monetization options that hold significant potential. This combination enhances both the utility and financial viability of the project.
We want to emphasize that all potential monetization strategies listed above are meant to increase the chances of sustaining and expanding the system. Despite that, our main objective remains a FREE and OPEN framework. If it's possible to sustain the project and provide all services listed above completely for FREE FOREVER, we definitely prefer to keep it this way. If you have any ideas on how this can be achieved, we'd be thankful for you sharing them with us. Your opinion is important to us. If you have any feedback on this matter, feel free to contact us and share your thoughts.
Future Plans:
In our ideal future vision, we aspire to create a comprehensive media manipulations detection tool that supports images, video and audio content analysis, evaluating combinations of text and images in articles, voice tonality in audio and video content, background images and video footage, as well as body language and facial expressions in video content. This represents the challenging goal of creating a process that considers all possible modalities and analyzes how they are integrated with each other in any piece of content, be it an article, book, podcast, or video.
Special thanks to:
1littlecoder, Yohei Nakajima, Matt Wolfe for the great inspiring content that made us fall in love with AI-powered apps. It was this inspiration that led us to create HonestyMeter, and we're grateful for their contribution!
Our heartfelt gratitude extends to the entire community of AI researchers whose groundbreaking work has been instrumental for our project. Without their dedication and creativity, HonestyMeter would have demanded an investment a thousand times larger and a team a hundred times bigger.
We give special recognition to OpenAI for their exceptional advancements in generative AI, which have been crucial in realizing our vision. Our personal thanks go to Sam Altman, Ilya Sutskever, Greg Brockman, Elon Musk, and all the other talented individuals who contributed to the development of this transformative technology. Their visionary leadership and commitment to innovation in AI have not only made our project achievable, but have also enabled thousands of other innovative projects, significantly advancing the frontiers of technological possibilities.
Important Considerations When Using the HonestyMeter Framework:
When using the tool for the first time, you may be shocked by the high levels of subjectivity even in the content of the most well-known and authoritative mass media sources. It is essential to acknowledge that no one can be entirely objective, and some degree of bias is inevitable. Furthermore, a low objectivity score does not necessarily indicate malicious intent on the part of the mass media or journalists. Many instances of biased content are created unknowingly, with the best of intentions.
Our goal is not to blame anyone, but to provide a valuable tool for content creators and consumers alike that can help improve objectivity in media content. By using the HonestyMeter framework thoughtfully and with an understanding of its limitations, we can take a step towards creating a more reliable and trustworthy source of information for all.
Conclusion:
The HonestyMeter framework has the potential to be a game-changer in addressing media bias and misinformation. It's widespread adoption could increase transparency and objectivity in mass media, by helping journalists and content creators to produce more objective content, empowering users to make informed decisions with ease and becoming an essential tool for anyone seeking truthful and unbiased information.
Join Us in Shaping the Future of Media Truth
Up to this day, HonestyMeter has been fully self-funded. We invest our own time and money in research, development, and maintenance. Though we are fully capable of progressing independently, we are open to the possibility of partnering with those who resonate with our vision and can offer a substantial contribution, whether it be enhancing visibility, funding collaborations, or offering expertise.
If you share our vision of truthful media and are interested in making a contribution that has the potential for major advancement, please feel free to reach out to us at info@honestymeter.com.
Together, we can let the truth triumph.
Honest Disclosure:
This text was evaluated by the HonestyMeter and found to be highly biased towards promoting mass media transparency and the use of the HonestyMeter. 😊